The challenges caused by blur when using widefield microscopy
Fluorescence microscopy is a powerful tool for answering a wide range of biological questions, especially when it comes to detecting, observing, and tracking specific cellular structures, molecules, and proteins. Depending on the application, microscopists using fluorescence will often turn to widefield microscopy.
A key benefit of widefield microscopy lies in its speed and sensitivity, making it very attractive for imaging dim or moving samples. Its main disadvantage is that the entire 3D volume is illuminated and captured, meaning that regions above and below the focal plane will also fluoresce, increasing background while also reducing contrast. The result can be a blurry image, which can render downstream analysis steps such as segmentation and annotation more time-consuming and prone to error, especially when dealing with thick samples. Given the overall advantages of widefield microscopy, any method that can minimize the impact of blur when analyzing thicker samples would be of great benefit. A solution to this is the use of Leica Microsystems’ camera-based THUNDER Imager, to remove background, in combination with Aivia software which allows for automated and accurate segmentation. We will discuss this synergy in more detail below.
Removing blur using advanced widefield imaging systems
THUNDER significantly reduces the impact of blur while Aivia improves the accuracy of THUNDERed image segmentation and analysis. This synergy is especially powerful when using thick or highly scattering specimens.
The THUNDER imaging system includes a computational clearing capability that removes the blur and haze typically seen in widefield imaging, allowing clear marking of signal and background during image segmentation.
The images captured by THUNDER also have better contrast than raw widefield images, which makes it easier to accurately segment cells and other objects of interest. This is where THUNDERed images really come into their own, as it is much easier and faster to train AI-driven software tools like Aivia to automatically segment objects when images have been THUNDERed. With Aivia, users get access to object-based boundary refinement, robust high-content informatics, adaptive image region partitioning and morphologic processing, all of which lead to accurate segmentation.
Analyzing immune cell populations during inflammation
A research project to investigate the distribution of immune cells in whole-mount organ specimens during inflammation showcases how THUNDER and Aivia can be used to improve the accuracy and speed of image analysis.
The current protocols for analyzing whole-mount organ samples are often elaborate, expensive, and organ-specific. To overcome these challenges, researchers at the Technical University of Munich in Germany developed a simple, cost-effective, non-hazardous, whole-mount approach for efficient tissue clearing and multi-organ volumetric imaging, which they call EMOVI [1]. Among other steps, the approach uses THUNDER to remove blur and Aivia to automatically segment the images. In this case, the combined solution was able to save the team a significant amount of time and effort,
especially as there was a large number of immune cells that needed to be marked and tracked in each specimen. Using EMOVI, the team were able to investigate the prevalence and location of immune cells in a variety of organs, even when using thick tissue samples.
As an example, the THUNDERed image below shows the distribution of immune cells in a section of white adipose tissue (left-hand image: blood vessels in pink, immune cells in white). The figure also includes the results of an automated analysis conducted using Aivia, where each immune cell was color-coded based on its distance to the nearest blood vessel (right-hand image). The EMOVI technique allowed the researchers to image more than a millimeter into the specimen, with THUNDER limiting the impact of blur and background to improve segmentation accuracy. In addition, when compared to manual image segmentation, Aivia made it much faster and easier to segment and analyze the hundreds of immune cells present in each image.
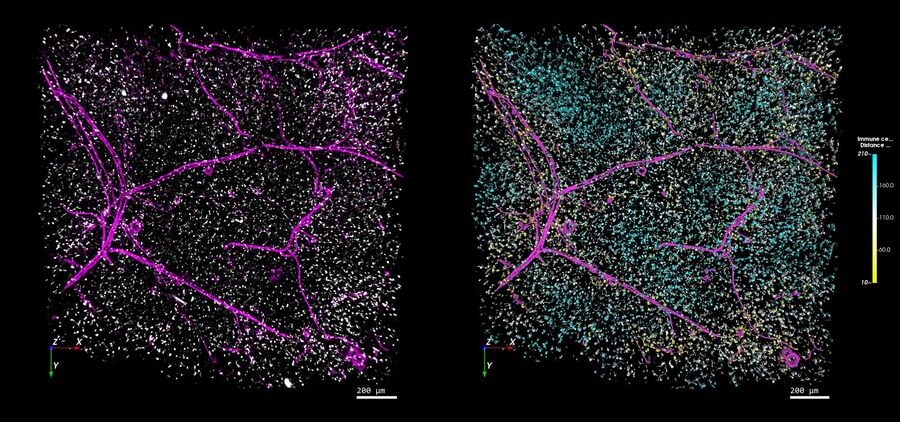
Trying to precisely analyze the samples in this study would have been challenging and time-consuming without access to the speed and accuracy benefits provided by THUNDER and Aivia. More widely, the research demonstrates how the EMOVI approach can be used to accurately assess the spatial distribution of cells, blood vessels and other structures using widefield fluorescence microscopy, even when working with thick, whole-mount samples.