Many methods exist to remove background noise, the most commonly used being rolling ball and sliding paraboloid [1,2]. Recently, Leica Microsystems introduced their own background subtraction method called Instant Computational Clearing (ICC) [3], which is present in all Leica THUNDER WF imaging platforms. Independent of the chosen background subtraction method, each algorithm strives to minimize noise and feature erosion while retaining the underlying structural details of the image. Most importantly, these background subtraction methods aim to improve the analysis and quantification of captured data.
Common background subtraction methods
Rolling ball (Figure 2C) is a common background subtraction algorithm that uses a structural element placed over the image with a radius of curvature of the ball set by the user in pixels [1,2,4]. To efficiently remove the background, the pixel radius is set to a pixel value that is as large or slightly greater than the largest sized feature in the image. Relative to the intensity peaks of the features in the image, the background is considered smooth, and with the structural element set to a value larger than the width of the feature, the structural element operates on the background by changing the local background value of the pixel. Often, a Gaussian filter is applied to the image prior to implementing the rolling ball, with the Gaussian filter acting as a low pass frequency filter that smoothens the image. Applying a Gaussian filter prior to the rolling ball can help to reduce image noise yielding an improved background subtraction.
Sliding paraboloid (Figure 2D) is another popular background subtraction method, similar to rolling ball. Sliding paraboloid replaces the ball with the apex of a paraboloid, with a radius value defining the curvature of the paraboloid [4]. By sliding the paraboloid across the image, the local background is subtracted by estimating the intensity variation across the apex. This method can be a better treatment of the data when the features do not correspond well to pixel values. Functionally, the subtracted background is performed in a similar way to rolling ball, and pre-filters such as Gaussian filtering can be applied to suppress noise in the resultant image.
Rolling ball, sliding paraboloid and other background subtraction methods require the use of structural elements, wavelets or point spread function modelling to estimate and subtract the background [1,2,4]. Identifying the parameters for efficient background removal with minimal feature erosion can be time consuming, is typically performed manually and implemented as a post-acquisition workflow. Leica Microsystems has developed a new background subtraction algorithm which addresses many of these challenges.
Leica’s solution: Instant Computational Clearing
The ICC algorithm [3] offered by Leica uses a different approach to increase image contrast. ICC does not implement structural elements, wavelet transforms, or use point spread function (PSF) modeling to estimate the background noise. ICC improves image contrast by considering the entire image and applies a model to distinguish signal (structures within the image) from the background noise. Similar to minimizing a cost function [5-8] for linear regression, ICC minimizes a non-quadratic cost function to estimate the background noise, Eq 1:

where y is the input parameter, x is the background noise to be estimated, γ‖∇x‖22 is the L2 regularization term, and γ is the regularization parameter. The regularization parameter is set to a default value proportional to the full width at half maximum of the PSF of the optical system or is a user selectable value based on the size of specific features in the image. Collectively, γ‖∇x‖22 acts to penalize the cost function to prevent overfitting of the background, or erosion of targeted features. Once the background noise has been estimated, it is subtracted from the image to reveal the true signal (Figure 2B).
Conclusion
Both rolling ball and sliding paraboloid can be found in 3rd party image analysis tools including paid microscopy software packages as well as open source software such as FIJI [4]. Variations do exist in how these subtraction methods are applied; however, they are commonly implemented on the raw data as a post-processing step outside of the imaging workflow, post-acquisition. The application of these two background subtraction methods on the raw data is an iterative process, requiring the user to identify the best pixel value to use to enhance the features in the image while preserving structural details. In contrast, ICC is fully integrated into the imaging workflow for background noise removal and image contrast enhancement. ICC can be applied both post-acquisition or during acquisition, the latter providing an instantaneous real-time preview of the contrast improved image as the data is being acquired. The unaltered raw data is preserved following ICC, which allows users to perform ground truth analysis. Following ICC, both the raw and the processed datasets can be further compared and analyzed using the 3D visualization and 2D and 3D analysis packages in Leica’s LASX software.
Image background subtraction using either rolling ball, sliding paraboloid or ICC produce images with improved clarity. However, the improvement is limited to an enhancement in contrast without any effect on image resolution. To improve image resolution Leica offers an adaptive deconvolution algorithm that can be paired with ICC to produce an image that has both improved contrast and resolution. The adaptive nature of the algorithm arises from the creation of adaptive signal to noise ratio coefficients on a voxel-by-voxel basis that constrains the deconvolution through regularization. An in-depth discussion of Leica’s adaptive deconvolution will be covered in our next upcoming technology brief.
Download the technical brief
Click here to download the technical brief as pdf.
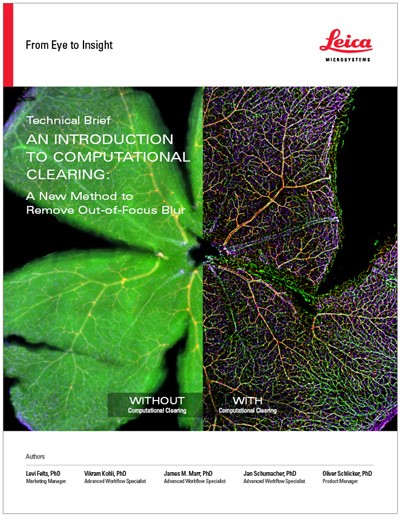